30 Feature Flagging Best Practices Mega Guide
Essential tips, tricks, definitions, use cases, and more to improve your feature management practice.
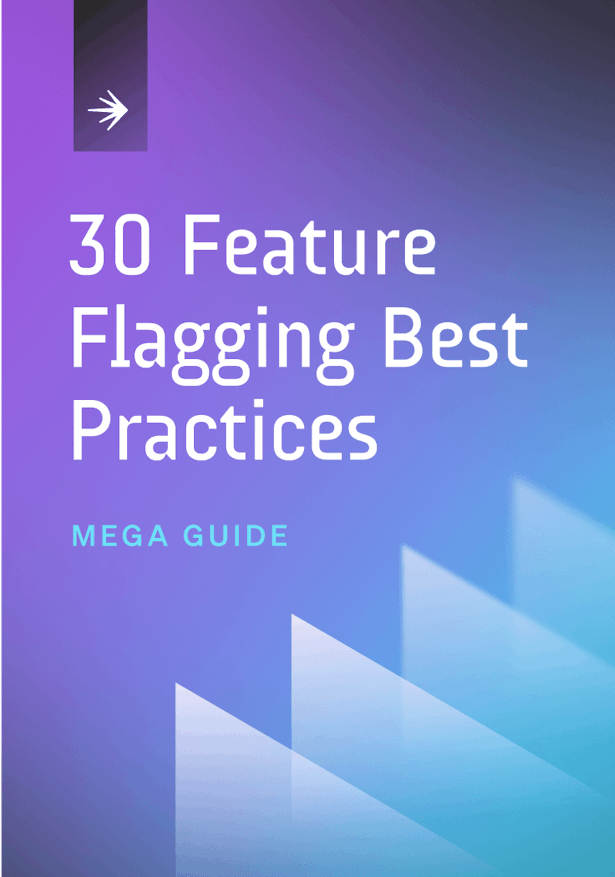
9 best practices for experimentation
What makes a good experiment?
Experiments can validate that your development teams’ efforts align with your business objectives. You can configure an experiment from any feature flag (front-end, back-end, or operational), but what makes a good experiment?
Experiments use metrics to validate or disprove gut feelings about whether a new feature is meeting customers’ expectations. With an experiment, you can create and test a hypothesis regarding any aspect of your feature development process, such as:
- Will adding white space to the page result in people spending more time on the site?
- Do alternate images lead to increased sales?
- Will adding sorting to the page significantly increase load times?
Experiments provide concrete reporting and measurements to ensure that you are launching the best version of a feature that positively impacts company metrics.
General experimentation best practices
Let’s first talk about best practices around creating an experiment. Even with a solid foundation of feature flagging in your organization, missteps in these areas can yield flawed results. Consider these best practices for experimentation.
1. Create a culture of experimentation
Experiments can help you prove or disprove a hypothesis, but only if you are willing to trust the outcome and not try to game the experiment. Creating a culture of experimentation means:
- People feel safe asking questions as well as questioning the answer. It’s ok to question the results and explore anomalies.
- Ideas are solicited from all team members—business stakeholders, data analysts, product managers, and developers.
- A data-driven approach is used to put metrics.
Part of creating a culture of experimentation is providing the tools and training to allow teams to test and validate their features.
Needed for experimentation:
- Tools to help you collect relevant metrics can include monitoring, observability, and business analytics tools
- Tools to help you segment users
- Tools to clean and analyze the results
2. Define what success looks like
Experiments help you determine when a feature is good enough to release. But how do you define what “good enough” is and who is involved in creating the definition? Experiments can involve a cross-functional team of people or only a handful, depending on the focus of the experiment. For example, if an experiment is focused on whether to add a “free trial” button to the home page, you may need to involve people from demand generation, design, and business development.
A good experiment requires well-defined and agreed-upon goals and metrics by all stakeholders. Ask yourself what success looks like. It should be defined as improving a specific metric by a specific amount. “Waiting to see what happens” is not a goal befitting a true experiment. Goals need to be concrete and avoid ambiguous words. And then, goals and metrics should be tied to business objectives like increasing revenue, time spent on a page, or growing the subscription base.
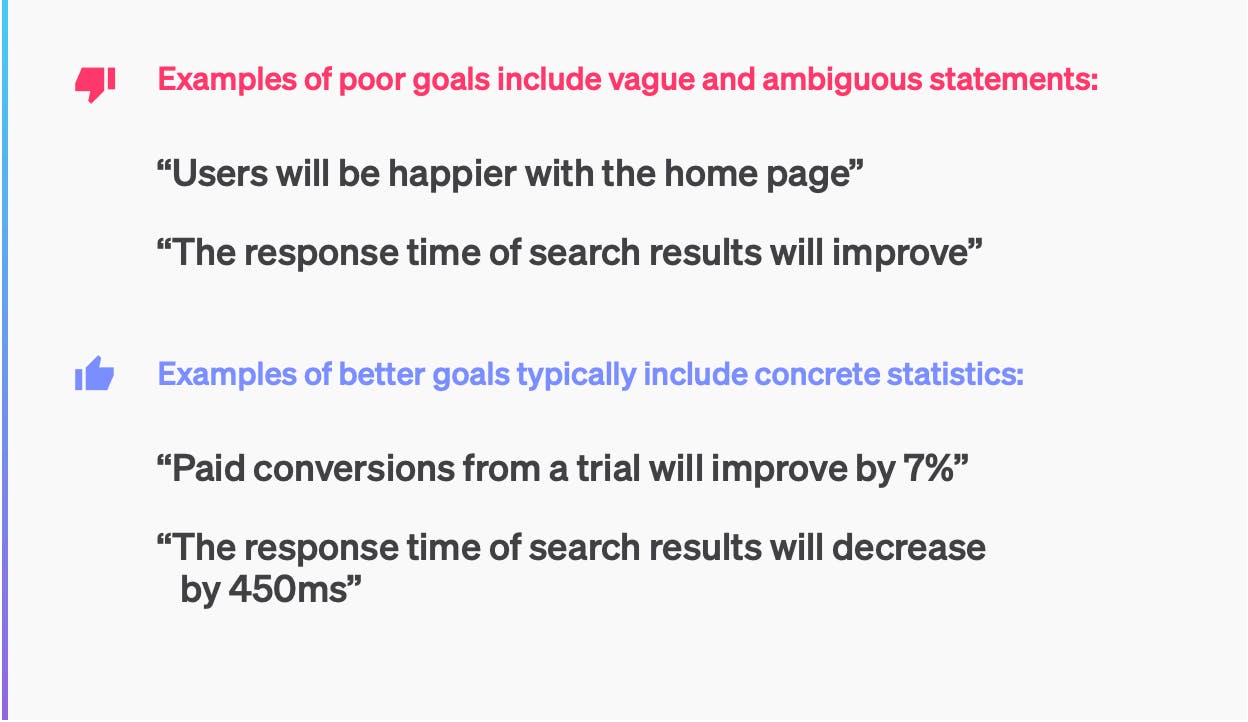
3. Statistical significance
Looking at a single metric is good, but viewing related metrics is even better. Identify complementary metrics and examine how they behave. If you get more new subscribers—that’s great, but you also want to look at the total number of subscribers. Let’s say you get additional subscribers, but it inadvertently causes existing subscribers to cancel. Your total number of subscribers may be down as a result, so does that make the experiment a success? Probably not.
The number of samples will depend on your weekly or monthly active users and your application. No matter the size of the samples, it is important to maintain a relatively equal sample size. Significantly more samples in one cohort can skew the results.
4. Proper segmentation
Think about how and when users access your application when starting an experiment. If users primarily use your application on the weekends, experiments should include those days. If users visit a site multiple times over the course of a couple of weeks before converting and before the experiment starts, early results may be skewed, showing a positive effect when it was neutral or negative.
There are two aspects to segmentation. First, how will you segment your users, and second, how will you segment the data? A successful experiment needs two or more cohorts. How you segment your users will vary based on your business and users. Some ways to segment users:
- Logged in vs. anonymous
- By company
- By geography
- Randomly
No matter how you segment users, always make sure the sample sizes are balanced. Having skewed cohorts will result in skewed results.
When analyzing the data after an experiment, you may want to do additional segmentation to see if the results vary based on other parameters.
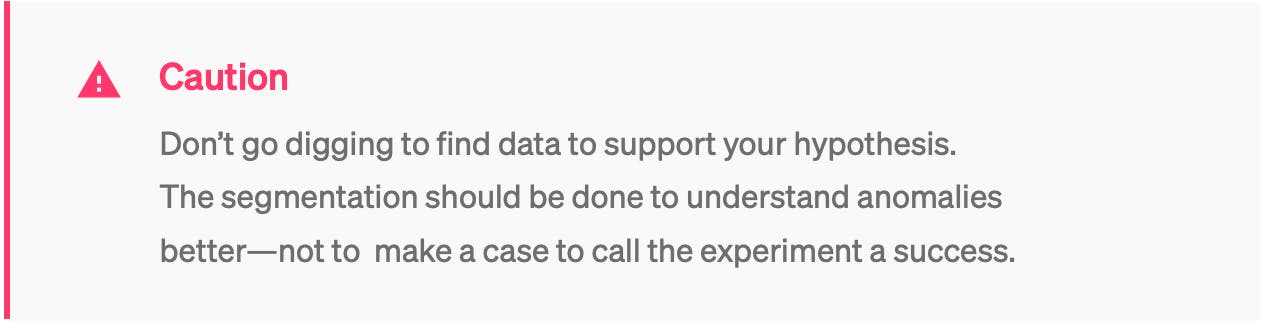
5. Recognize your bias
Everyone is biased. This isn’t necessarily a bad thing; it is simply a matter of how our brains work. Understanding your biases and those of others will help when running experiments. Our biases influence how we process information, make decisions, and form judgments.
Some biases that may creep in:
- Anchoring bias - our tendency to rely on the first piece of information we receive
- Confirmation bias - searching for information that confirms our beliefs, preconceptions, or hypotheses
- Default effect - the tendency to favor the default option when presented with choices
- The bias blind spot - our belief that we are less biased than others
It is common in an experiment to scrub the data to remove outliers. This is acceptable, but make sure you aren’t eliminating outliers due to bias. Don’t invalidate the results of your experiment by removing data points that don’t fit the story
6. Conduct a retrospective
Experiments are about learning, so hold a retrospective after each one. Questions to ask can include:
- Was the experiment a success? Did we get the results we expected? Why or why not?
- What questions were raised by the experiment?
- What questions were answered?
- What did we learn?
And most importantly, should we launch the feature? You can still decide to launch a feature if the results of an experiment were neutral.
Experimentation feature flags best practices
Now that we’ve covered the basics of experiment best practices, let’s dive into best practices around using feature flags in your experiment. Well-executed feature flagging is a foundation for a well-run experiment.
If you’ve embraced feature management as part of your teams’ DNA, any group that wants to run an experiment can quickly do so without involving engineering.
Follow these best practices to get the most from your existing feature flags:
7. Consider experiments during the planning phase
The decision of whether to wrap a feature in a flag begins during the planning phase— which is also the right time to think about experiments. When creating a feature, evaluate the metrics that will indicate whether the feature is a success: clicks, page load time, registrations, sales, etc.
Talk with the various stakeholders to determine whether an experiment may be necessary and provide them with the essential information on the flag, such as the name of the flag, to configure an experiment.
8. Empower others
When giving others the ability to run experiments, make sure you have the proper controls in place to avoid flags accidentally being changed or deleted. Empower other teams to create targeting rules, define segments, and configure roll-out percentages while preventing them from toggling or deleting flags.
9. Avoid technical debt
Experimentation flags should be short-lived. This means removing a flag after an experiment completes and is either rolled out to 100% of users or not released. Put processes and reminders in place to remove the flag.